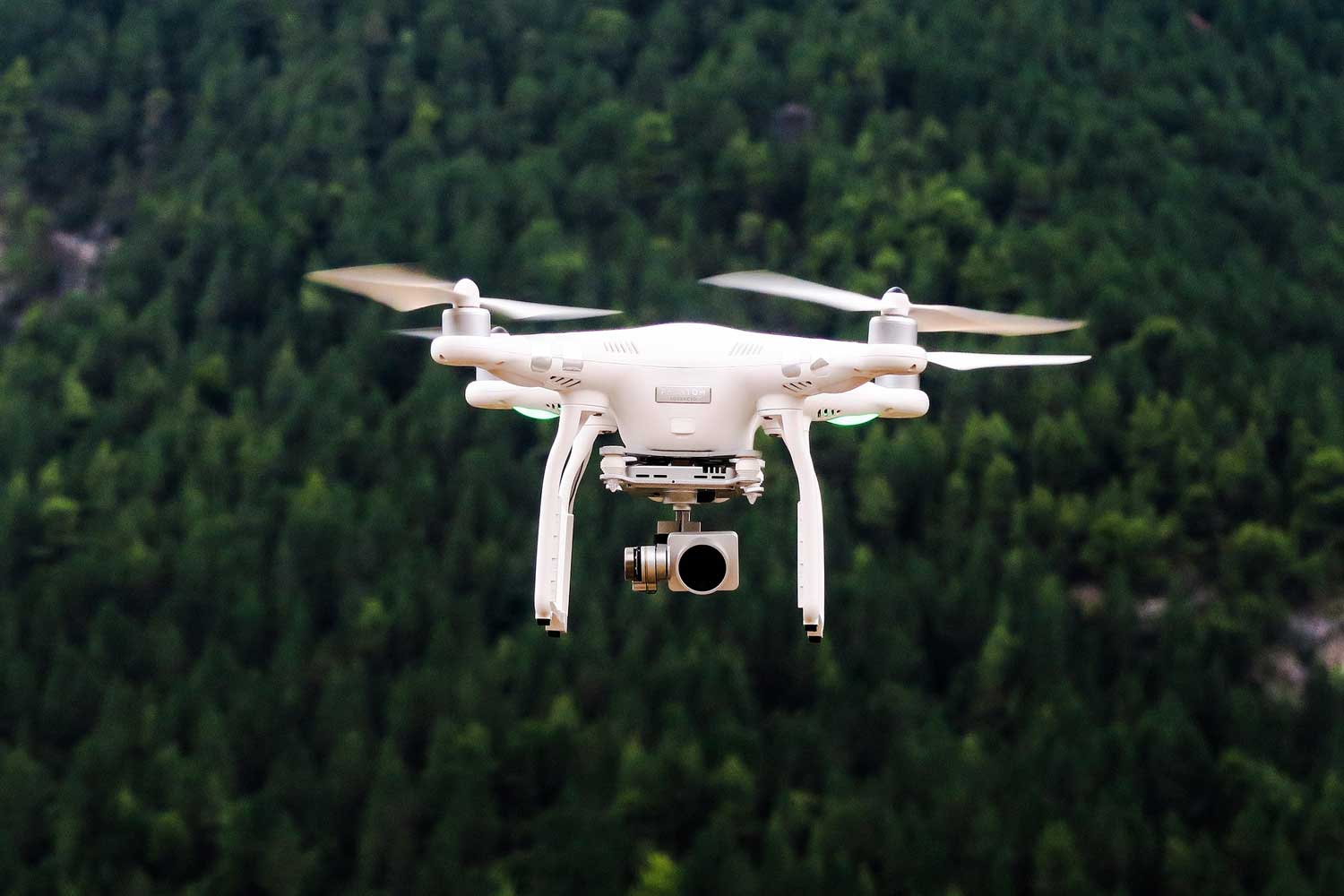
Fighting wildlife crime with AI drones
An AI-driven drone that processes data onboard—eliminating the need for a remote control center—enables real-time surveillance, instant threat detection, and rapid response to poachers, can help to prevent wildlife crime.
Fighting Wildlife Crime with AI
Artificial intelligence (AI) is playing a pivotal role in tackling the global poaching crisis, transforming how we defend endangered species in reserves across Africa, Asia, and South America. Together with fixed cameras on the ground, an AI-driven drone-based system can provide real-time surveillance and rapid response capabilities against poachers.
Drones: Aerial Guardians in the Fight Against Poaching
Conservationists often face an uphill struggle when patrolling vast, isolated terrains where poachers operate undetected. Similar to fixed cameras like TrailGuard AI that monitor animals at ground level, drones can monitor huge swathes of land far more swiftly than rangers on foot. However, currently, many drones capture footage and send it back to control centres for AI analysis.
In contrast, the Eco-Watch Guardian project explores an alternative approach using AI software to process data directly on the drone. This onboard processing enables real-time threat detection, allowing the drone to identify potential poachers immediately.
Developed as a research prototype by the team at Sathyabama Institute of Science and Technology in Chennai, India, Eco-Watch Guardian illustrates how AI technology can be used to protect vulnerable wildlife.
Inspiration from UAV Guidance Technology
The inspiration for Eco-Watch Guardian drones stems from insights gained from advances in UAV guidance that leverage deep learning for real-time object detection and tracking.
A key element in this technology is YOLO (You Only Look Once), a fast and efficient object detection algorithm that processes an entire image in one go, making it ideal for rapid identification in UAV applications. Complementing YOLO, object monitors continuously track detected objects across successive frames, ensuring consistent identification even if a target temporarily disappears or changes appearance. While YOLO excels at ultra-fast processing, resource-constrained devices such as drones require a balance between speed and accuracy.
Therefore, Eco-Watch Guardian employs MobileNet SSD—which combines the lightweight MobileNet architecture as a feature extractor with the Single Shot Detector (SSD) framework—to achieve this optimal balance for real-time detection of wildlife threats. The SSD framework streamlines detection by generating predictions in a single pass, making it particularly suitable for real-time applications on drones.
The Single Shot Detector (SSD) framework is a deep learning-based object detection model designed to detect objects in real-time. Unlike traditional models that scan images in multiple stages, SSD generates predictions for objects directly within a single pass, or "shot," through the network. It works by dividing the image into a grid and generating bounding box predictions and class probabilities for each part of the image simultaneously. This single-step approach makes SSD faster and more efficient, making it particularly suitable for resource-constrained environments, such as drones.
At the heart of Eco-Watch Guardian, this lightweight yet powerful CNN detects humans or vehicles hidden under thick foliage using specialised sensors like high-resolution cameras or thermal imaging. Onboard processing allows the system to analyse data in real time, and as soon as suspicious activity is flagged, the drone sends an immediate alert—often within seconds—to ranger teams via GSM, long-range radio, or satellite links. This rapid notification can make the difference between intercepting poachers and arriving too late to prevent harm to wildlife.
How the Eco-Watch Guardian System Works
- Data Collection and Training
Much like other AI-driven solutions, Eco-Watch Guardian relies on massive datasets, including images gathered from drones, camera traps, and open-access wildlife databases. These datasets are meticulously cleaned, annotated, and occasionally augmented (e.g. flipped or rotated) so that the underlying AI can cope with diverse lighting conditions, terrains, and weather patterns. - AI-Enhanced Identification
Having been trained on large volumes of labelled data, MobileNet SSD is then fine-tuned to spot poachers, suspicious vehicles, and even particular animal species. By honing its detection capabilities, the system drastically reduces false alarms—an essential feature when rangers must deploy resources quickly and efficiently. - Onboard Processing
A standout feature of Eco-Watch Guardian drones is their ability to analyse video footage onboard, without relying on a stable internet connection. This means they can detect threats in real time, even in remote regions lacking mobile coverage. In some scenarios, where more intensive analytics might be necessary, footage is sent to a central server or command centre for further processing. - Geospatial Precision
When a potential threat is identified, the system immediately tags it with GPS data, guiding rangers straight to the source of the alert. This level of geospatial precision proves indispensable when operating in immense reserves, where every minute spent tracking down poachers matters. - Adaptive Learning
Over time, Eco-Watch Guardian continues to refine its detection accuracy by learning from previous mistakes—whether it’s a rustling bush mistaken for a person, or a legitimate visitor mislabelled as a poacher. These iterative improvements ensure the drones become even more reliable with every deployment.
Strength in Numbers
Much like having dozens or even hundreds of fixed cameras in strategic places, deploying multiple drones could scale up a reserve’s protection exponentially. Even a small team of rangers can gain the equivalent coverage of far larger forces simply by launching a fleet of AI-powered drones. That level of responsiveness is invaluable in remote locales or extensive parks, where poachers have traditionally taken advantage of limited manpower.
Conclusion
From protecting elephants and rhinos in Africa to safeguarding tigers in Asia, AI-powered drone surveillance could revolutionise wildlife conservation. A future where a network of AI-driven drones provides a comprehensive shield over vulnerable ecosystems would make it increasingly difficult for poachers to operate undetected.
As more reserves consider integrating artificial intelligence into their anti-poaching strategies, there is renewed hope that threatened species can be preserved—before it’s too late.
References (downloads)